Hypotheses
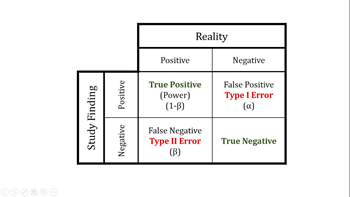
- Null hypothesis (H0)
- hypothesis of no difference
- e.g., there is no link between disease and risk factor
- hypothesis of no difference
- Alternative hypothesis (H1)
- hypothesis of difference
- e.g., there is a link between disease and risk factor
Type I Error (False Positive)
- Stating there is an association when none exits
- incorrectly rejecting null hypothesis
- α = probability of type I error
- p = probability that results as or more extreme than those of the study would be observed if the null hypothesis were true
- general rule of thumb is that statistical significance is reached if p < 0.05
Type II Error (False Negative)
Power (True Positive)
- Probability of correctly rejecting null hypothesis
- Power depends on
- size of expected effect
- increasing effect size increases power
True Negative
- Probability of correctly accepting null hypothesis
Confidence Interval
- Range of values associated with a confidence level indicating the likelihood that the true population value of a parameter falls within that range
- usually done with 95% confidence interval (2 standard deviations from the mean)
- e.g., based on our study data, we are 95% confident that the average salary of a teacher lies between $30,000-45,000/year
- Confidence interval is calculated from statistics generated from the studied data
- Smaller confidence intervals suggest better precision of the data
- Larger confidence intervals suggest less precision of the data
- If confidence intervals of 2 groups overlap, there is no statistically significant difference
A Priori Versus Post Hoc Analysis
- A priori comparisons
- comparisons planned prior to data analysis
- planning dependent on knowledge researchers have prior to conducting statistical tests
- Post hoc analysis
- researcher decides additional comparisons to make after viewing data
- choices dependent on knowledge researchers have gained after conducting statistical tests
- e.g., a test is run that says there is a difference between groups A, B, and C
- post hoc analysis would involve comparing group A to group B, B to C, and A to C to see between which groups the difference lies
- one potential hazard is an increased likelihood of spurious statistical associations
- e.g., a test is run that says there is a difference between groups A, B, and C